Beyond Collection: Building Publicly Available Information Systems for Strategic Effect
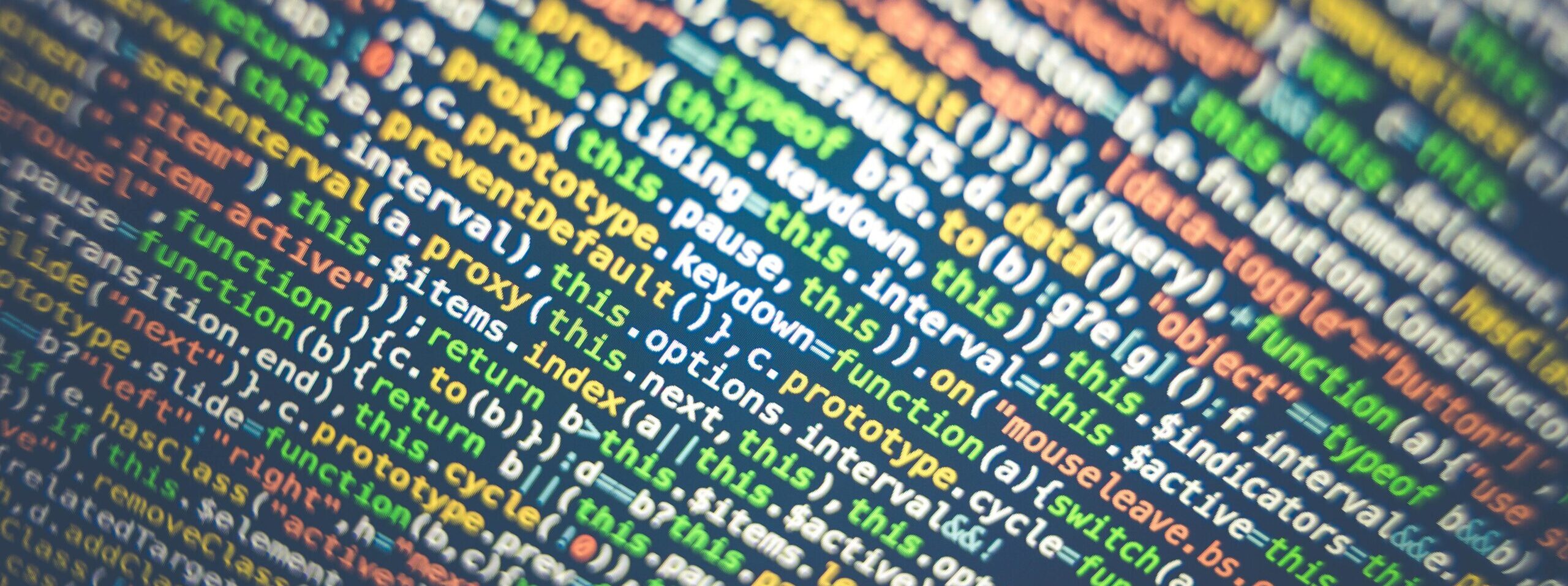
Unaffiliated Researcher: Jessica Dawson
The U.S. Army recently published a new Information doctrine. For an organization that is often resistant to change, publishing a doctrine on information is a monumental change. However, it is also a necessary change; recent conflicts show that information has become a critical aspect of all modern warfare. This dimension encompasses content and data, and analytical and technical processes used to exchange information across operational environments. Just as each operational environment is multifaceted and complex, so too is the information dimension. A particularly complex, yet important component of the information dimension of the operational environment is Publicly Available Information (PAI), or open-source information.[1]
PAI is an increasingly critical source of information for military operations. While exact numbers are not known, significant amounts of classified intelligence used to drive operations come from PAI. Furthermore, PAI is the most important medium for information warfare and controlling narratives. PAI is an essential source of battlefield intelligence and operational assessment. And, as battlefields become increasingly digitized, the importance of PAI to military operations is likely to grow.
Despite the new doctrine and the importance of PAI, the U.S. Army is still unequipped to understand – let alone dominate – the information dimension of warfare. Current PAI analytic tools are insufficient for military understanding of the informational dimensions of the operational environment. With the growing complexity and value of the informational dimensions, the problems with current PAI analytic tools will only worsen. In this article, we outline limitations of current PAI tools, brought on by the nature of the information dimension itself as well as the technical considerations for analyzing digital information. We will then present a new paradigm for how PAI analysis tools should be made to support military operations. Overall, PAI analysis tools need to shift from the current offerings that are primarily based in dashboards with no access to data, to designs which are interoperable and focus on data acquisition and availability. These analytic tools need to prioritize greater variety in data collection, the ability to access that data programmatically, and user-level configurability of tools over niche machine learning models and visualizations on dashboards.
Changes to The Information Dimension
To understand the necessary evolution of PAI analysis tools, it is crucial to dissect why current offerings fall short. Current PAI tools currently fail in three, main ways: they do not allow access to data, they are not designed for military use, and they are not customizable. To understand why these are critical shortcomings, we first must understand the nature of the information dimension.
The information dimension is complex, voluminous, and dynamic – and largely indecipherable to nonspecialists. This results in two things. First, it means that military analysts struggle to distill digital information into actionable intelligence for a commander to make more informed decisions. Second, it is never clear what information is important for any given operational context. Furthermore, most commercial PAI tools are Commercial-off-the-shelf (COTS) products designed for advertising, brand management, and consumer analytics. Thus, the information from these tools, which is typically only presented as a dashboard (i.e., a visual computer interface that presents key data and metrics in an organized format), is of limited military value.
Additionally, the social media environment, one of the most important parts of the information dimension, has become more fragmented and complex. There are now dozens, if not hundreds, of different social media sites with varying structures and rules. For example, many sites now have some form of private or semi-private page or channel for certain types of conversations. Some sites only work with images and videos for posting, while others allow only text. This means that certain analyses will be important for one particular social media site but useless for others. Additionally, it means that the analysis of social media data must extend beyond text to other modalities. The changing nature of the social media environment will necessitate that military analysts be able to analyze multiple modalities within different contexts due to the different governing rules of different social media sites.
Furthermore, useful PAI in the military context will come from more than just Western social media sites. As social media fragments, there is an increasing number of foreign, non-English language social media sites and subdomains within social media sites. These foreign, relatively-small social media sites can provide very useful information for military operations. However, most commercial PAI analysis offerings only focus on Western social media outlets for data collection and only have tools for handling English. So, most commercial PAI analysis offerings have a significant data gap.
Additionally, to get more complete PAI analyses, analysts need access to more than social media data. Legacy sources of PAI, such as traditional print media, online websites, commercial data, and others also provide operationally important information. This presents a future where data streams of various types of PAI will need to be configured at low levels of military operations. This requirement is unique and unless the military drives the demand for these small market efforts, the commercial demand signal will be insufficient to create a market opportunity. At present, current PAI tools frequently do not have foreign social media data, non-social media data – and most importantly – completely lack the ability to integrate new streams of data at an analyst level.
Why Are Current PAI Tools So Ineffective?
Current PAI tools generally seek to condense a lot of technical information down to manageable information displays in a particular context. The vast majority of current PAI tools are a combination of some unknown collection of social media data combined with usually unspecified machine learning (ML) models and a dashboard. All a user can interact with is that dashboard. Unless it so happens that a particular PAI tool happens to have the correct data and analysis for a given operational context, the tool will likely be unable to address PAI analysis needs.
It is this latter point which makes even those PAI analytic tools actually designed for military use come up short. The nature of what constitutes militarily relevant analysis in the information dimension for any given operation is continually evolving and poorly understood. Truly leveraging PAI requires multiple tools, and every operational context will need different tools. Emerging disciplines like social cybersecurity, which didn’t exist a decade ago, have come into existence because whole new aspects of the information dimension are now becoming militarily relevant in new and unforeseen ways. The information dimension, and what aspects are militarily relevant to it, are continually changing. Thus, even at a conceptual level it is not possible to create one dashboard that will be sufficient for military PAI analysis. It is this point that has continued to plague the authors when analyzing PAI for military commanders and the main reason for writing this piece; every PAI tool we have tried to use in a military context is never able to answer all of the questions that a commander has. This indicates we need a new paradigm for PAI tools.
Since these tools do not allow access to the underlying data, it’s also impossible to customize analyses to support a particular operational context. For example, a military organization may care about things like detecting bots or coordinated inauthentic behavior, but any given PAI analysis tool only has dashboard displays for things like trending topics and aggregate sentiment scores. And, without access to underlying data, it becomes impossible to do any analysis outside of that dashboard. Furthermore, without access to the underlying data it’s not clear how representative any results presented by the dashboard actually are; if all one can see are aggregate statistics, one cannot know if these aggregate statistics describe the whole environment or just some specially selected subset of it. The fact that these tools do not allow access to data forces users to make assumptions about what can be seen in the dashboards (e.g., using sentiment as a proxy for opinion or stance) and leaves many military analysts of PAI unable to fully understand the information dimension and advise commanders.
The nature of how machine learning models operate contributes to the inadequacy of current dashboard-only tools. As PAI consists of a plethora of sites and voluminous data streams, detailed analysis must leverage machine scalability in order to draw useful insights from it. This means that innovations like machine learning are critical to the analysis of PAI , particularly to deal with the sheer magnitude of data that is available. However, these models are also often fragile, and require maintenance to remain current and deliver correct results. Furthermore, current machine learning models are often specially designed for a particular task, like sentiment classification or bot detection. Thus, when a user is only presented with a dashboard, they cannot maintain or create specialty ML models which reduces the usefulness of the PAI tool. Finally, researchers and ML developers are constantly creating newer, better models as well as models for new tasks in the information dimension. All of this means that a PAI tool must allow for changing and updating any ML models, or other analysis methods, at the user level, or the tool will fail to keep pace with operational demands and remain relevant.
Changes to Technology
Recent technologies provide the possibility for new types of PAI analysis tools and methods. Groundbreaking models like Large Language Models (LLMs) and Vision Language Models (VLMs) are ushering in a new era where analysts can dynamically create ML algorithms tailored to their specific analysis needs. Unlike the current generation of PAI offerings where analysts must adapt their tasks to fit available ML tools, Foundational Models empower them to instead adapt ML tools to their tasks. For example, using LLMs eliminates the need to settle for current PAI offerings, like generic sentiment algorithms, when trying to analyze complex socio-linguistic concepts like opinions on specific events or individuals.
An analyst can instruct an LLM to directly perform these operationally-specific analyses using everyday language. Analysts can also craft custom, high-performing algorithms in real-time, leveraging model distillation and data programming, which overcomes the limitations of pre-packaged, narrow tools prevalent as currently offered. These narrowly-focused, opaquely-trained ML models packaged with current PAI analysis tools are nowhere near as performant or flexible as using Foundational Models outside of those tools.
A New Paradigm
While new technologies can address some issues with current PAI tool sets at the analyst level, certain aspects in the information dimension are better handled at the tool-maker level. Data acquisition, especially for social media data, remains a persistent challenge. Social media sites frequently change rules on data collection, adjusting technical methods like APIs and data formats with the introduction or removal of features. This dynamic landscape makes acquiring internet data, assessing that data, and integrating that data with other sources or databases an ongoing challenge. Due to the increasing intricacy of the information dimension in online environments, evaluating data quality and linking together relevant data pose ongoing challenges. Solving these data-related technical issues often requires a high degree of expertise in niche skill sets like web scraping, which are not easily trainable. Addressing challenges related to data acquisition and integration demands specialized knowledge, and the evolving nature of the online landscape ensures that expertise in areas like web scraping remains crucial for effective tool development and maintenance.
With all the current shortcomings and changes in the information dimension, it’s clear that the military doesn’t just need new PAI tools but rather a new paradigm where the analyst is central and tools and data are customizable to them. PAI tools should fundamentally focus on interoperability and data acquisition, as opposed to dashboarding and ML models. Organizations should be able to access all collected data from a vendor. Analysts should be able to query any number of PAI tools from a programming environment to combine data sources and ML models to create customized analysis pipelines. The age of full suites with just access to a dashboard for an analyst is over, and having a special algorithm for a niche concept is no longer a selling point for a PAI tool.
PAI tools need to be configurable at all levels, from data inputs to the construction of analyses. This paradigm of configurable and modular PAI tools fits into a growing need to have such digital tools across all warfighting functions. Current PAI offerings for military personnel resemble buying a gas station sandwich: average bread, meat, cheese, and vegetables all in one package — or nothing. PAI offerings should be more like getting a sandwich in a market, with choices between exquisite offerings of bread, meat, cheese, and more, allowing users to assemble data and analyses on demand. PAI tool makers should stop focusing efforts on creating mediocre, full suites and instead concentrate on creating exquisite capabilities in critical aspects like data acquisition or foundation models.
Figure 1: Comparison between current and future paradigms for analysis tools for Publicly Available Information (PAI). Graphic created by the Authors.
An important implication of this paradigm shift is that PAI analyst training will also need to adapt to using foundational models and basic data science skills. While not everyone in the military information dimension community (i.e., OSINT, PAO, PSYOPs, etc.) needs to be able to deep dive into PAI analytic tools and data, more of the force does need to overcome the fear of handling data. Also, many of the skills required to use digital data and operate things like foundational models are very trainable, making it not unrealistic for more of the force to utilize PAI data. At the same time, leaders need to better understand what PAI analysis can tell them about a military problem and, potentially, how to solve it. The data, PAI tools and the understanding they can bring aren’t as confusing or complicated as many people have made it out to be. The military should continue to push for operators, analysts and leaders to be trained to manipulate and understand digital data. In an era where information is as vital as ammunition, equipping ourselves with adaptable, customizable PAI tools is not just a necessity but a strategic imperative to excel in modern warfare.
[1] For the purpose of this article, we utilize Army doctrinal terms. The informational dimensions of the operational environment are human, information and physical. We will collectively refer to these three dimensions as the ‘informational dimensions’.
The views expressed are those of the authors and contributor, and do not reflect the official policy or position of the Department of Defense or the U.S. Government.