SWJ Book Review – Understanding Dark Networks: A Strategic Framework for the Use of Social Network Analysis
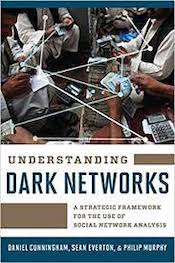
SWJ Book Review – Understanding Dark Networks: A Strategic Framework for the Use of Social Network Analysis
Isaac Poritzky
Daniel Cunningham, Sean Everton, and Philip Murphy, Understanding Dark Networks: A Strategic Framework for the Use of Social Network Analysis. Lanham: Rowman & Littlefield Publishers, 2016. [ISBN 978-1442249448, Paperback, 388 pages]
Understanding Dark Networks provides an introduction to Social Network Analysis (SNA), a relatively new, and underutilized research technique that provides a view of dark networks unachievable in traditional lenses. SNA “can be used to gain a greater understanding of dark networks as well as craft strategies that undermine them” (p. xvii).
The authors, Daniel Cunningham, Associate Faculty for Instruction at the Common Operational Research Environment (CORE) Lab embedded in the Naval Postgraduate School’s Department of Defense Analysis, Sean Everton, Professor in the Department of Defense Analysis and the Co-Director of the CORE Lab, and Philip Murphy, Associate Professor at Middlebury Institute of International Studies at Monterey are experienced scholars who have written extensively on “dark networks” and run the CORE lab, whose mission it is to serve as the leading Department of Defense (DOD) organization in providing research and education to US and foreign military officers in advanced methodologies and cutting-edge, analytic technologies. SNA is the next essential methodology for DOD analysts, practitioners, and researchers.
Background on SNA
Valdis Krebs’ (2002) seminal analysis of the 9/11 hijacker network[1] along with Marc Sageman’s (2004) study of 172 Islamic terrorist operatives[2], and many other studies, have laid the groundwork for the function and utility of SNA be it qualitative, quantitative, or mixed methods in security studies.
Critics of SNA argue that the three main challenges are “the dynamic and evolving nature of networks, the incompleteness of the data, and the fuzziness of boundaries” (p. xviii). Cunningham, Everton, and Murphy argue that the main issue is not in finding dark network data, but sorting through it. Even with these challenges, SNA enhances our understanding of dark networks and potential strategies for disruption.
The majority of SNA research focuses on the usefulness for identifying key players whose removal from the network would disrupt it. That is one use, but Understanding Dark Networks raises the awareness of the wide range of metrics available and the various corresponding strategies. More so, it addresses how SNA can assist a myriad of kinetic (mainly targeting) and non-kinetic (tracking or monitoring, information operations, rehabilitate or reintegrate, and institution building) approaches to the tracking and disruption of dark networks.
Even in its simplest form, sociograms or visualized networks, SNA offers a powerful new perspective for those with an already solid foundation of a dark network. When more complicated quantitative analysis methods are undertaken—e.g., centralization and brokerage measures—SNA can explain a variety of behaviors due to its tendency to force researchers “to think in terms of constraints and options that are inherent in the way social relations are organized.”[3]
What truly separates SNA from other traditional quantitative approaches (i.e. frequentist statistics which focus on a random sample of actors and their attributes) is that SNA focuses on patterns that affect behavior. Concurrently, Cunningham mentions that analysts of dark networks often limit their focus to attributes, which can cause incorrect assumptions. An actor holding a mid-level position in a terrorist group is unlikely to gain significant attention due to their lack of formal prestige, but they may be a successful recruiter or key mentor for many lower-ranking members. SNA acts on the key concept that “actors do not make decisions autonomously; people are substantially influenced by the behavior and choices of other actors around them” (p. 14). SNA allows researchers to transcend expected attributes and understand dark networks for what they really are: a group of complex social interactions and relationships that accounts for empirical context.
Structure of the Book
Understanding Dark Networks is split in four sections. The first section consists of three chapters that introduce readers to the assumptions, uses, collection, coding, and manipulation of social network data. Chapter 1 examines SNA and how it differs from other quantitative methods. It also exposes readers to basic SNA terms and concepts so that they will not become lost in later chapters.
The second section builds on the first section and explains the uses of several “families” of metrics commonly used for exploratory network analysis. Chapter 4 introduces readers to algorithms that identify a network’s central actors. Chapter 5, positional analysis, introduces readers to algorithms that identify actors holding similar positions, e.g., the chief surgeons of different hospitals.
The third section consists of three chapters that focus on confirmatory techniques for examining dark networks. Chapter 9 focuses on hypothesis testing techniques for analyzing dark networks, mainly quadratic assignment procedure (QAP) and conditional uniform graphs (CUGs). Chapter 10 introduces exponential random graph models, which provide analysts with a way to examine the internal (endogenous) and external (exogenous) social processes. Chapter 11 examines methods for understanding changes in a dark network over time. The book concludes with a chapter that summarizes key items and lessons learned on which future research can build.
In general, Understanding Dark Networks functions as a textbook that provides basic explanations along with instructions on how to embark on several quantitative methods. Cunningham et al, focus on UCINET, but also address Net-Draw, Pajek, ORA, R, and Gephi. There is a significant lack of focus on Gephi, a popular software package, so this book would be most helpful for researchers interested in learning or growing their skills with UCINET. There are numerous images captured from these software packages that provide visuals to assist in understanding SNA. Along with the helpful images, the authors created tables that list the pros/cons, uses, interpretations, and potential caveats of different measurements. These tables are by far the most practical and useful addition to the book.
Understanding Dark Networks and SNA, in general, are an essential tool for the study of dark networks and criminal insurgency. Francisco Sollano (2022) conducted a SNA of Genaro García Luna, the former head of Mexican Federal law enforcement, and his ties to the Sinaloa Cartel [4]. This study helped to better understand the corrupt Mexican officials allowing illegal trafficking of drugs inside Mexico and into the United States. Sollano’s which cited Cunningham et al and Everton’s disrupting dark networks, study built on Jones et al’s (2020) social network analysis of the Tijuana Cartel where he studied the “internal structures and the roles of individuals in cross-border polydrug networks under pressures from rivals and state leadership targeting” [5]. Dr. Jones was able to use SNA to understand the relationship between actor centrality and network topography, along with location of criminal activity, and location of residence. These studies are only the beginning of the usefulness of SNA.
Conclusion
Cunningham, Everton, and Murphy’s book is most relevant for practitioners, researchers, or anyone seeking to understand dark networks. Practitioners often worry that SNA is difficult to learn and comprehend, but through Cunningham’s easily accessible writing, anyone can understand and apply it after reading this book.Understanding Dark Networks would also have utility for teaching SNA to undergraduates, graduate, postgraduate students in many fields. The early chapters are accessible to anyone, but the last section requires baseline statistical knowledge.
Social Network Analysis represents the future of dark network analysis, ranging from jihadist groups to gangs. All intelligence analysts and researchers would see their research value increase with an in-depth understanding of SNA. Understanding Dark Networks: A Strategic Framework for the Use of Social Network Analysis should be an essential read for any practitioner or security scholar interested in learning or furthering their knowledge of SNA.
Endnotes
[1] Valdis Krebs, “Mapping Networks of Terrorist Cells.” Connections. Vol. 24, no. 3. 2002: pp. 43-52, https://www.aclu.org/sites/default/files/field_document/ACLURM002810.pdf.
[2] Marc Sageman, Understanding Terror Networks. Philadelphia: University of Pennsylvania Press, 2004.
[3] Jörg Raab and H. Brinton Milward, “Dark Networks as Problems.” Journal of Public Administration Research and Theory. Vol. 13, no. 4. 2003: p. 417, https://doi.org/10.1093/jpart/mug029.
[4] Francisco Sollano Jr, “A Social Network Analysis of Genaro García Luna and His Alleged Ties to the Sinaloa Cartel.” Small Wars Journal. 11 January, 2022, https://smallwarsjournal.com/jrnl/art/social-network-analysis-genaro-garcia-luna-and-his-alleged-ties-sinaloa-cartel.
[5] Nathan Jones, W. Layne Dittmann, Jun Wu, and Tyler Reese, “A Mixed Methods Social Network Analysis of a Cross-Border Drug Network: The Fernando Sanchez Organization (FSO).” Trends in Organized Crime. Vol. 23, 2020: pp. 154–182, https://link.springer.com/article/10.1007/s12117-018-9352-9.
[6] Sean Everton, Disrupting Dark Networks. Cambridge: Cambridge University Press, 2012.