Developing a Big Data Strategy: “Where We Are” and “Where We Need to Go”
Ingrid Parker
The world is on the cusp of an epochal shift from an industrial to an information-based society. History demonstrates that changes of this magnitude do not occur without being accompanied by fundamental change in the way war is conducted. This “Information Revolution” is a product of advances in computerized information and telecommunications technologies and related innovations in management and organizational theory. (Davis, 1996, p. 79)
Recognizing Transformation: Revolutionary Military Affairs (RMA)
Throughout our military history, innovation caused adaptations on the battlefield that have been remarkable and innovative. We call these adaptations Revolutionary Military Affairs (RMA). The easiest technological adaptation (or RMA) to recognize is the repeating rifle, patented in 1860 by Benjamin Tyler Henry. The repeating rifle caused armies to consider standoff, cover and concealment, and new maneuver in their tactical formations. Prior to the repeating rifle, armies fought mostly using Napoleonic formations; however, the accuracy of the repeating rifle, caused a shift in maneuver on the battlefield. Another adaptation, which is less noticeable, but just as important, is the shift from courier reporting to radio reporting, with the introduction of the radio into tactical formations during World War I (WWI). The technological innovations of WWI marks it “as the first modern war, since a number of technological inventions made their debut during the war” (Lallanilla, 2014. para 1). Like the repeating rifle, the technological innovations in WWI caused new tactics on the battlefield to include rigid reporting techniques like scheduled reporting, standard formats, a common language, and taxonomy. Commanders and other leaders still used face-to-face communications and battlefield circulation as the main method to grapple with situational awareness, however, reporting was designed to augment and improve battlefield visualization.
This RMA or technological transformation occurred in the summer of 1914, when Germany conducted a hasty military mobilization for an impending war in Europe. The Chief of the German General Staff [or the Chief of Staff (CoS) of the German Army], Helmuth von Moltke the Younger, understood that he prepared his Army for a war that had unpredictable outcomes due to military overmatch and a war-plan with a non-contiguous, battle-space strategy famously called the Schlieffen Plan. As directed by the Kaiser (Wilhelm II), Moltke readied the military for war; even though, he believed that the plan was too audacious in operational reach and force array. That said, the Schlieffen Plan was renowned and well-liked by the Kaiser and field commanders because it contained political objectives that made it very desirable to the military and national leaders alike. Moltke, however, was concerned about the plans’ assumptions regarding timing, tempo, and the political objectives, as they were ill-defined and overly audacious.
Prior to this hasty mobilization, Moltke wanted to aggressively revamp the Schlieffen Plan because he did not think his Army was ready to execute a two-front war with Russia and France. In addition, he recognized that the plan assumed (or predicted) that the British would not intervene in the conflict and he was not sure this was a valid assumption. Moreover, the Schlieffen Plan had no room for error and it did not account for the political conditions (changes in the international balance of power) nor technological innovations of the day.
In 1914, several subordinate Army commanders put the Schlieffen Plan into motion by going on the offensive at the Battle of the Frontiers. Likewise, Moltke’s follow-on offensive actions and choices reinforced the plan, making it a maneuver decision and battlefield reality. After the Schlieffen Plan was enacted, Moltke struggled to gain and maintain situational awareness throughout the depth of the battle-space due to poor battlefield visualization and ineffective operational reach in the range and depth of the battlefield. Historians often assert that Moltke weakened the Schlieffen Plan by amassing forces on the left side and diminishing the right side; thus, he did not set the conditions for a French Army defeat (Zuber, 1999).
To assist with information management, which Moltke believed was the heart of the problem, he implemented rigid reporting techniques, mostly field reports to better understand the battlefield. The German Army predominantly used courier as the means for battlefield visualization; however, this quickly became obsolete on the non-contiguous battlefield due to the implementation of radios. Nevertheless, Moltke had neither the staff, nor staff-expertise, to conduct thorough analyses of the reports, as the reporting came into the command center. Consequently, information arrived, but went unevaluated when it came to decision-making. Despite his only partial view of the battlefield, Moltke often assessed the situation and made decisions based-on conjecture in the theater of war. His inability to see the battlefield in depth often caused him to make poor use of military resources in terms of decisive and culmination points (Bendikowski, 2016, p. 2). In retrospect, Moltke believed that the reports had the information and answers that he needed; however, it was still inaccessible due to his inability to manage and organize the information. In the case of the Great War, Moltke was not able to see (or visualize) the battlefield in depth, causing campaigns to become disjointed. In the end, he lost operational control and subsequently the war. I believe the German Army did not fully adapt to the volume of reporting generated by radio communications, which would have improved battlefield visualization and timely battlefield decision-making.
During the interwar period, the German Army’s leadership conducted a rigorous After-Action Review (AAR). To address some of the challenges they faced in the Great War, the new Chief of Staff, Hans von Seeckt, revamped the Army’s staff, doctrine, training and tactics (Bendikowski, 2016, p. 2). He implemented new organizational hierarchies for modern warfighting and for adaptation to air-land battle. More importantly, he recognized that telecommunications (the radio) made the courier obsolete and, therefore, continued to refine the reporting structures and reporting mechanisms that emerged under Helmuth von Moltke the Younger. He, too, understood that he was in effect readying the German Army for another war - World War II (WWII) (Bendikowski, 2016, p. 2). This paradigm shift and RMA (from courier to reporting) occurred in the early 20th century (1914-1926) because of the German Army’s inability to manage the volume of information and ineffective staff processes for the emergent technologies during the Great War. The new reporting methodologies effectively supported new military technologies, information needs, and the new modes of warfighting, as tested by WWII.
Although the German Army lost the Second World War, they had mastered information management with the new reporting techniques and methodologies. In a roundabout way and through AAR, the United States (U.S.) Army and the Department of Defense (DoD) adopted the German Army’s business practices and incorporated similar principles into its doctrine, training, organizational force structure and reporting schemas. Over the next few decades, reporting permeated all facets of U.S. government and remains the primary deliverable for intellectual exchange and knowledge production. Like the German Army, the U.S. Army, DoD and the U.S. government still use these reporting techniques today; even though, technologies, information and warfighting have since adapted to the information age, again.
U.S. Army and the DoD Adaptation
While it is true that the U.S. Army, DoD and the intelligence community have adapted the force structures and information systems over the last few decades, it was not due to the exigency of information nor unlimited data. The nature of war was the driver, causing data management to be an afterthought and quite ad hoc in its implementation. Like in 1914, we are at the crossroads of technology and data availability, causing analysts and organizational leadership to function in the middle of a technological RMA as seen in mixed business practices (modern and antiquated work processes); therefore, we must adapt our Army and intelligence apparatus to meet the demands of information, intelligence, and speed. As an organization, we must implement Big Data management processes to augment and improve battlefield visualization, organizational decision-making and to free up analyst time, similar to the decisions made between the years 1914-1926, the first information RMA.
The Fundamentals of Data Management
Housekeeping
Housekeeping is the process of record-keeping, maintenance, and other routine tasks that must be completed in order for an ecosystem to function efficiently and succinctly. Housekeeping occurs in tools and services such as databases, system processes, core services, and interfaces. Although mundane, often overlooked and under-executed; housekeeping is a necessary function for Big Data implementation – very much like processing, exploitation, and dissemination (PED) is to the intelligence cycle. In addition, core services must be implemented to emulate and embed intelligence authorities – the following list is a sample list of core services that would be applied broadly in the Big Data intelligence environment.
- Purge and recall-ability for data provenance
- Foreignness determination for E.O. 12333
- Serialization of bulk data for auto dissemination
- Entity disambiguation and entity resolution for enrichment
- Correlation, merge and canalization for data curation
Understanding Emergent Intelligence Tasks
As in the Moltke example above, von Seeckt understood that staffs were the necessary adaptation to create an analytical and a response function in the German Army in order to manage the flow of information as well as afford decision-making. However, data has evolved exponentially, and staff hierarchies are no longer the viable solution (or adaptation). Additionally, corporate America has already implemented Big Data methodologies in micro- and macro-marketing strategies, enabling decision-making in motion; greater agility and increased stakeholder participation; micro and macro consumerism; and targeted information campaigns. In order to maintain pace, adaptation is necessary and will come; however, adaptation will require new intelligence tasks. Below is a summary of the tasks that I anticipate.
- Back-end work is the hidden side of a technology or tool. This activity is often done on databases and servers or functions such as cataloguing or indexing. This type of work creates a technological foundation, a security apparatus, and the necessary content to be leveraged on the front-end (Pluralsight, 2015). A good example of back-end work is Project Maven.
- Front-end work is the user interface and user experience (Pluralsight, 2015). Brilliant technologists create a Graphic User Interface (GUI) that mimics human learning models, as it requires less training. The best example of a GUI that requires little training with high payoff is Google Maps. It is a multi-data analytic (or App) that offers decision-making to customers while on-the-move. In addition, it now uses customers to catalogue and index through their route selections and Google searches; hence, Google has automated back-end work too.
- “Machine learning is an application of artificial intelligence (AI) that provides systems the ability to automatically learn and improve from experience without being explicitly programmed” (Zulanas, 2017, para. 5). “Machine learning focuses on the development of computer programs that can access data” while simultaneously learning from it (Zulanas, 2017, para. 5). AI uses TTP mapping, enabled from hierarchical tools, analyst documentation, and algorithms - meaning it must be able to replicate analyst decisions, as seen with IBM’s Watson.
- Databasing is a systematized and hierarchal collection of data that can be accessed immediately and manipulated by data processing systems for a specific purpose (Free Dictionary, 2018). Usually, databasing involves maintaining records composed of hierarchal fields that contain information about a particular phenomenon. Databasing enables machine learning and AI because it contains organized information that AI can understand. In addition, technologists can then create and construct algorithms that emulate the human thought process in areas such as indications and warnings, merges/correlations, and anomaly detections. For intelligence activities, databasing will include adding information to specified data fields, structured reporting, entity enrichment, transliteration, and the implementation of ontological standards.
- Web scraping is the process of extracting targeted information from the internet and storing it locally (Yee, 2016). Scrapers extract information from WebPages, PDFs, or other openly available websites in order to make it useable for further processing or data enrichment. Entity and topic scraping are used for purposes such as (1) the enrichment of order of battle and identity intelligence, (2) Chi-Square for anomalies, and (3) trend analysis.
- A data management strategy is necessary in order to plan or create a long-term plan for handling data that is “created, stored, managed, and processed by an organization” (Techopedia, 2018, para. 1). “It is an IT governance process too that aims to create and implement a well-planned approach for organizational data assets” and data equities (Techopedia, 2018, para. 2). Our data organizational strategy should include front-end classification tagging, some front-end processing, automated dissemination, geo-tagging, and data modeling.
Getting Back to the Basics
To start new intelligence tasks, organizations must concentrate on the core competencies [collection by Area of Intelligence Responsibility (AOIR), PED, analysis, and intelligence synchronization] rather than complicated details or new theories. This means the intelligence apparatus must be optimized against the commander’s priority intelligence requirements (PIR). As such, the intelligence apparatus must focus on PIR-SIR-SOR-SDR and the associated reporting mechanisms (e.g. IIRs, TACREPs, RECCEEXREPs, KLIEGLIGHTS, INTREPS, CEMs, GEOINT reports, searchable PDFs, annotated PowerPoints, textual reports, and OSINT word reports). Point-to-point intelligence dissemination (email, in person, or briefings) is a nicety, but it is time-intensive; thus, leaders must tightly manage point-to-point dissemination to improve efficiencies. Consequently, optimizing delivery is as necessary as clean business practices, too. Below is a list of data methodologies that increase efficiencies or lay foundational processes to be leveraged later, using analytics, Apps, interfaces, or statistics.
- Develop Word Repositories: First, word data is the intelligence community’s staple and basic product. Word data is commensurate to transactional data in corporate America, which is used for micro and macro-marketing. When in stored in All-Source repositories, word data can be used for anomaly detection by instantiating Apps that simply use Chi-Square. In addition, long-term historical analysis can be used by creating phenomenon baselines – for example, the number of extra judicial killings by month and by threat entity. This information can then be examined in context of current governmental activities, which is often the impetus for increase or decrease in this activity. Examples of word repositories are iSight and High Point.
- Clean Processes: Go back to the basics on Area of Intelligence Responsibility (AOIR) and Intel Handover Lines (IHL), Enforce PIR-SIR-SOR-SDR linkages and Feedback Loops, Optimize the Intel Cycle, Manage PIR-IR to the Ability to Process/Collect, etc. Ensure we use the intelligence system of record; even though, new intelligence business practices will likely conduct analysis outside of the suite, using High Point or Agency tools. Then, interfaces must be developed for DCGS-A to receive that intelligence from these kinds of tools, extending its relevancy, too.
- Clean Data: “The process of detecting and correcting (or removing) corrupt or inaccurate records from a record set, table, or database and refers to identifying incomplete, incorrect, inaccurate or irrelevant parts of the data and then replacing, modifying, or deleting the dirty or coarse data” (Kosyakov, 2017, para. 2).
- Manage Organizational Technologies and Initiatives: Annually murder board initiatives and technologies and fund ONLY those that are moving the ball forward. Appoint technological Czar for governance, policy management, and technology funding/perpetuation. Allow for disruptive technological pilots, as they challenge status quo and force technological adaptation.
Interfacing in the Intelligence System of Record, DCGS-A
To make these types of systems and/or tools work seamlessly, interfaces must be developed to create bridges between technologies. Interfaces are independent and often-unrelated services that enable “disparate” systems to communicate, interact, and build upon each other’s capabilities. Interfaces require the movement of information multiple ways; therefore, proprietary systems and/or tools pose a problem for interfaces, as proprietary systems limit the movement of information, usually in unidirectional way. As such, proprietary technologies inhibit true cross-collaboration and communication because it usually only “receives” data and often “does not share” its own data. Businesses design proprietary business strategies to dominate and control the technology market, as well as extend the life of a particular technology. For leaders, understanding proprietarism is critical in order to make technological decisions, such as, interfaces, system features, Apps, and future growth.
To demonstrate cross-collaboration and communication strategy with fully developed interfaces, the proposed strategy (Figure 1) shows four distinct systems and/or tools with one addition, a Government Off the Shelf Solution (GOTS) for databasing (with its own interface). This schematic is the proposed solution for the 470th Military Intelligence Brigade and our unique mission set. In the diagram, the interfaces (between the technologies) are RSS Feeds (e.g. for enrichment), DCGS-A Integration Backbone (DIB) [e.g. for dissemination], the Cross Domain Solution Suite (CDSS) [e.g. to create data flows], Oracle’s iStore [e.g. to receive data flows], and a GOTS interface for databasing [e.g. for backend work]. These interfaces create (1) finished intelligence; (2) dissemination methodologies; (3) venues for customer consumption; (4) a Common Intelligence Picture (CIP); and (5) other Common Operating Pictures (COP), too.
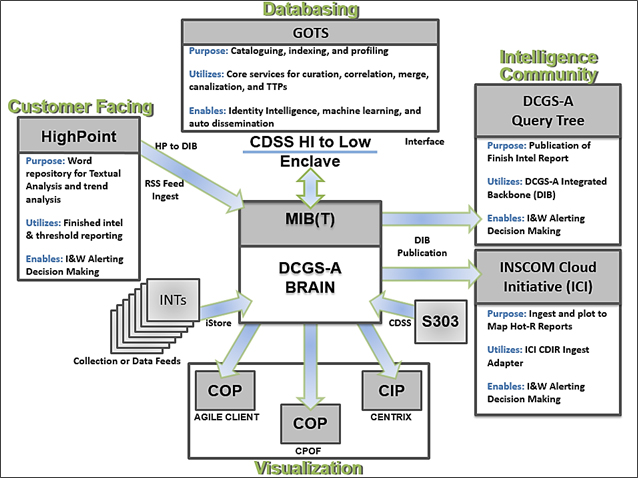
Figure 1. Proposed Information Flow in the 470th Military Intelligence Brigade
Source. 470th Military Intelligence Brigade, 2018
Know Your Data
Understanding the purpose behind data allows developers to plan repositories too. I particularly like cloud repositories or repositories that house metadata and word data together, so that advanced analytics (Apps) may be applied later. Optimal repositories have a GUI that mimics how consumers receive information from News Outlets and Social Media, which reduces training and maximizes consumer consumption and understandability. In addition, it creates relevancy from the onset while new features are iteratively added. Shown below are the types of data and their purposes:

Big Data Decision-Making
The most common decision-making model for data management is the Responsibility Assignment Matrix (RACI Matrix). Essentially, it manages risk by determining who has organizational authority (Doglione, 2016). This methodology marries up perfectly with AR 600-20 that assigns legal and intelligence authorities to commanders, unless subsequently delegated. Common questions in this framework includes “(1) Responsible – Who is completing the task?; (2) Accountable – Who is making decisions and taking actions on the tasks?; (3) Consulted – Who will be communicated with regarding decisions and tasks?; and (4) Informed – Who will be updated on decisions and actions during the project?” (Doglione, 2016, para. 2). Although simplistic, it retains the legal framework necessary to define the decision-makers, the doers, and the associated responsibilities.
- Decision-Making: For what purpose, how much to keep/purge, and where to house the data.
- Determine Co-Dependencies: Linking, geos/technologies, INT collection, databases, and database access, etc.
- Define Data by Purpose: Order of Battle - people, place, and things (database and analyst work), targeting products, lead generation, customer products, etc.
Conclusion
As an intelligence organization, we must consider data management processes including “acquiring, validating, storing, protecting, and processing required data to ensure the accessibility, reliability, and timeliness of the data for its users” and organizational stakeholders (NG Data, 2017, para 1). More importantly, we need a long-term strategy that organizes, makes sense of, and applies analytics to raw data for real-time, military decision-making, better customer engagement, and critical insights to threat steams. Optimally, our data management strategy would include data cleaning, storage considerations by purpose, the implementation of core services, a suite of web-based tools (or apps) for analytical work, and the introduction of new intelligence tasks. These tasks would formalize products that are wedged between intelligence analysis and PED - products such as geos, tips, raw data, running estimates, and targeting products. In addition, I would implement server virtualization that has a tier system in place (5Ws and how the data is hosted). Second, create a unified management process, perhaps an open management standard, to differentiate purpose in the data environment. Planners must understand intelligence storage needs as well as the purpose of storage in their planning efforts. Of course, we want a cloud architecture, but “how, why, and the amount of data” are critical decisions for organizational leaders – and these are probably the decisions of the day, as compared to von Seeckt staff additions for information management, fusion, and decision-making or Moltke’s reporting methods for battlefield visualization.
As stated above, there are various purposes for storage; therefore, we must determine the reason behind storage needs, as the first consideration because it is critical in the Big Data environment. To understand data storage, I like to offer our homes as a good analogy in order to comprehend it. For example, the bedroom closets are used to house clothes, shoes, and other personal items. In the Big Data environment, this would be our outward facing solutions, determining how we best represent the information/intelligence - smarter GUIs, better consumer and analyst consumption, and superior ease-of-use. Another example is the kitchen, which stores and houses food to nourish the human body. This type of storage would equate to healthy, expandable, and standardized datasets. We would nourish and expand this data by adding content and protecting it through compartmentalization, data access controls, double security standards (hard and soft certifications) and behavior network analytics. Finally, we would standardize kitchen-like data through the process of data modeling. This activity would build the structural foundation, using a unified management process. The last room in a home that has storage considerations is the bathroom. The bathroom removes excrement in an efficient and systematic way. It’s designed to remove body excretion to free the home of disease and vermin. Because of this, bathrooms have become fundamental part of every home. In the Big Data environment, planners must understand “what to retain and what to throw away” for the purposes of recall, purge, and data provenance. To begin the planning efforts, I would use the “salute” report for storage, extraction, and structured reporting because it offers a good starting point and has become the basis of spot reports, micro-targeting, and running estimates. I would start our data modeling efforts, using all elements of the “salute” report, and perhaps few other characteristics. By retaining the characteristics of a “salute” report, we would be implementing minimal storage strategy (approximately 38kB per record); however, this strategy offers a good building block for databases as well as expandable datasets for advanced analytics, too. In addition, salute-format extraction (and storage) is exponential and offers bang-for-buck for intelligence operations.
References
Bendikowski, T. (2016). Moltke: The Fallen Chief of Staff. Deutsche Welle, October 2016: 2.
Davis, N. (1996). “An Information-Based Revolution in Military Affairs.” Strategic Review, Vol. 24, No. 1, Winter, 43-53, U.S. Strategic Institute. Retrieved from http://www.rand.org/content/dam/ rand/pubs/monograph_reports/MR880/MR880.ch4.pdf.
Doglione, C. (July 25, 2016). Understanding Responsibility Assignment Matrix (RACI Matrix). Best Project Management Software Reviews 2018. Retrieved from https://project-management.com/understanding-responsibility-assignment-matrix-raci-matrix/.
Free Dictionary (2018). Database. Retrieved from https://www.thefreedictionary.com/databasing.
Henry Inc. (2017). Henry History. Retrieved from http://www.henryusa.com/henry-history/.
Kosyakov, I. (April 2017). Decision Tree for Enterprise Information Management (EIM). Business Excellence. Retrieved from https://biz-excellence.com/2017/04/17/eim/.
Lallanilla, M. (2014). The Science of World War I: Communications. Live Science Contributor. Retrieved from www.livescience.com/45641-science-of-world-war-i-communications.html.
NG Data. (2017). What is Data Management? Retrieved from https://www.ngdata.com/what-is-data-management/.
Pluralsight LLC. (January 28, 2015). What's the Difference Between the Front-End and Back-End? Retrieved from https://www.pluralsight.com/blog/film-games/whats-difference-front-end-back-end.
Techopedia Inc. (2018). Data Management Strategy. Retrieved from https://www.techopedia.com/definition/30194/data-management-strategy.
Yee, Jonathan A. (March 2016). Characterizing Crowd Participation and Productivity of Foldit Through Web Scraping, Naval Postgraduate School. Retrieved from https://calhoun.nps.edu/handle/10945/48499.
Zuber, T. (1999). The Schlieffen Plan Reconsidered. War in History, 6 (3): 262-306.
Zulanas, C. (2017). Strategic Transformation. Business Transformation Institute MSS. Retrieved from https://mssbti.com/youre-being-disrupted/.
About the Author(s)
Comments
I think that every business…
I think that every business owner who is thinking about growing their business asks these questions, and your goals and plans really make a big difference to your business strategy. I believe that you definitely cannot do without various software and applications now, so you can contact Erised https://www.erised.io/ to consult with developers and find the best solution for your business.